At What Age are Workers too Old?
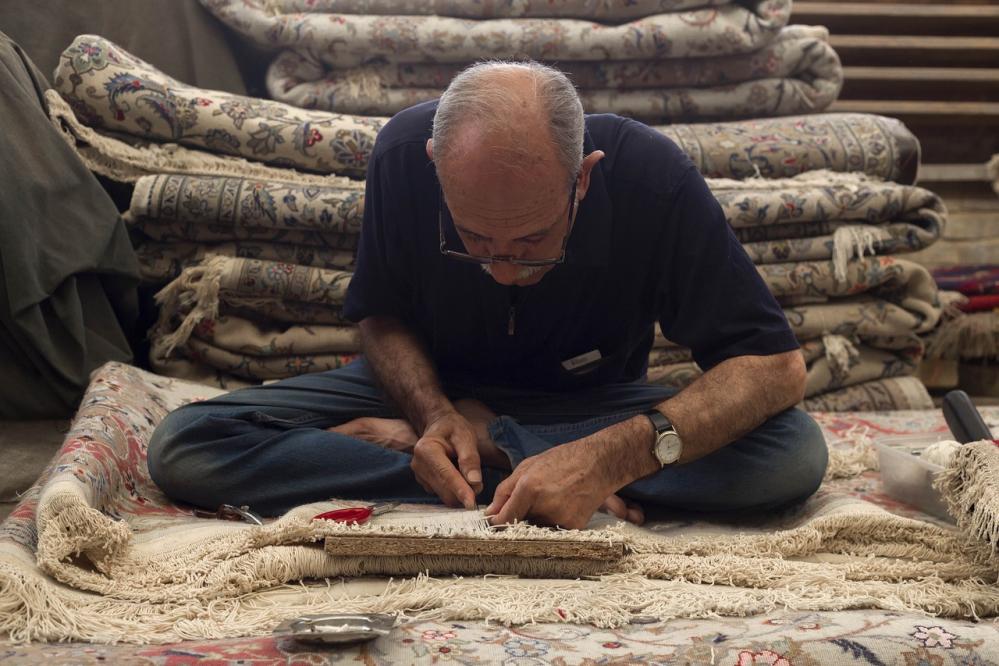
An article in the NYTimes https://www.nytimes.com/2023/06/28/business/china-jobs-age-discrimination-35.html noted that Chinese workers over the age of 35 are considered to be less job worthy than those under that age. This may come as a bit of a shock for Americans, long-accustomed to protection (often ineffective) from the Age Discrimination in Employment Act (ADEA) that prohibits discrimination against those age 40 and above https://www.dol.gov/general/topic/discrimination/agedisc . However, it does raise both theoretical and practical questions about how best to manage an aging workforce.
My early work in the field of cognitive aging focused on understanding the age-skill trade-off, particularly in the context of expert performance, often using chess playing as a model task to analyze. The context for the work was the relatively gloomy finding in large cross-sectional samples that many cognitive abilities showed linear age decline from the 20s onward. Similar but less steep decline trends were also found in longitudinal studies. These “fluid” abilities included working memory (memory for holding and manipulating information), spatial ability, reasoning, and particularly, speed of processing. The exceptions were “crystallized” abilities that tended to show increases from the 20s to the 50s or 60s and then slow decline thereafter. Acquired knowledge such as vocabulary and information commonly available in one’s culture fell into the crystallized category.
When thinking about job performance, both ability types are important: fluid, for novel problem-solving situations where you can’t immediately retrieve the solution, and crystallized, acquired job-related knowledge enabling a solution to be recognized immediately. Notably, such abilities can trade off, such that one type can substitute for another. When faced with a job-related problem, you could find a solution either by computing it on the fly, or by retrieving the answer from memory. Take the following simple problem that you are asked to solve mentally: “what is 95 x 95. You could apply the multiplication algorithm that you learned in public school and work your way through to the answer, making heavy use of working memory capacity to remember and store the partial products you need to generate the final answer. Or you could retrieve the answer if you have that specific problem stored in memory much as you learned the times tables for solving less complex arithmetic problems such as 9 x 9. You can get to the correct answer both ways.
In my research, I was looking at how age, which degraded fluid abilities that were important in searching for the best chess move, and acquired skill, which in chess was strongly related to the number and size of chess patterns you could quickly encode, traded off to predict performance. For some types of chess positions, you can recognize the correct move to make based on your internal representation of the patterns present in the position without having to search through a sequence of potential moves and countermoves. The knowledge-computation trade-off explained why chess players peaked in performance in their 30s and 40s rather than in their 20s as might be expected from fluid ability age curves. Humans can only do very limited searches through the huge tree of potential moves to consider from a given chess position. They use knowledge about patterns that are linked to plausible move candidates to choose a very small set of promising moves to explore through search. Knowledge constrains search, but it takes a long time (decade or more) to build up an expert pattern “vocabulary”.
So, depending on the nature of the mix of abilities that a job demands, you might be well suited to your job in your 70s (politicians?) or washed up in your late 50s (air traffic controllers must retire by age 56 in the US). Also, jobs that depend heavily on physical ability may not be sustainable much beyond the 50s (miners) though physical labor demands can often be reduced with the proper tools and environments. Particularly for knowledge workers, acquired knowledge can compensate for reduced computational abilities. We can also see a case for how artificial intelligence (e.g., large-language models such as ChatGPT) could support knowledge work. There are many other factors that weigh into a manager’s decision about hiring or retaining workers, beyond their skill level (unaided or aided). Older workers are more costly to insure (health) than younger ones and typically are paid more money (peak earning age is usually age 50-64 in the US). Age discrimination can be driven by many factors. However, ever-improving technology that can augment or substitute for waning capabilities may enable many of us to stay in the paid labor force much longer at higher levels of productivity.